In part one of this series, we took a look at the recently released Big Ten Men’s Basketball conference schedule and did some math to see how easy or difficult Michigan State’s schedule is compared to the competition. In general, MSU’s strength of schedule is in the middle of the pack. Among the projected contenders, MSU’s schedule is easier than Iowa’s schedule, but more challenging than Wisconsin’s and Illinois’ schedules.
While this information is interesting, the real question is what can the data tell us about MSU’s odds to hang a fourth consecutive regular season championship banner in the rafters of Breslin Center? Fortunately, I have just to tools at my disposal to answer that question.
I have described my methodology in the past in some detail, but the basic details are as follows. I start with the data from Ken Pomeroy’s website which tabulates the adjusted offensive and defensive efficiencies of each team. If these numbers are subtracted, it provides an “adjusted efficiency margin”, which correlates very well to the Las Vegas spread.
I have shown previously how the Las Vegas line correlates to the probability that the favored team will win straight up. The odds can be modeled using the the Normal/Gaussian distribution where the mean is the spread itself and the standard deviation is 10 points. I even derived why the standard deviation is 10 points, which is due to the fact that basketball teams usually shoot around 50 percent from the field and college basketball games usually consist of about 70 possessions. For reference, Figure 1 below shows the correlation between the spread and the odds for the favored team to win.
Figure 1: Correlation between the closing Vegas line and the odds that the favored team wins straight up. The best fit line is based on the Normal Distribution where the standard deviation is equal to 10.04 points.
Once there is a method to predict the odds for the outcome of any arbitrary game, it is possible to set up a Monte Carlo simulation to calculate the odds of various full season outcomes. This type of simulation is basically a very large set of virtual coin flips using a random number generator where the “coin” is weighted based on the odds generated from the Kenpom efficiency margin data and the correlation in Figure 1.
In this case, I simulated the entire Big Ten regular season a full 1,000,000 times, because my computer didn’t have anything better to do on a Friday night. The results of this simulation provide a great deal of information, including the expected number of wins for each team, as well as the odds of winning anywhere from zero to 20 conference games. Those data are summarized below in Table 1.
Table 1: Expected win totals and the full win distribution matrix based on 1,000,000 Monte Carlo simulations of the Big Ten season utilizing Kenpom's preseason adjusted efficiency margin data
As mentioned in Part One, the preseason Kenpom data suggests a very competitive Big Ten race, with six Big Ten teams in Kenpom’s preseason top 20 and 10 Big Ten teams in the preseason top 30. As such, the top 10 conference teams all have similar expected win totals which range from just over 10 win for Minnesota and Indiana to over 13 wins for Wisconsin.
Not only do the Badgers have the highest preseason Kenpom ranking, but they also have the easiest overall conference schedule. As a result their projected expected win total (13.3) is over a full game higher than the next two teams on the list: Ohio State (12.0) and Michigan State (12.0).
As for MSU, as the win distribution data shows, based on this analysis the Spartans are most likely (53 percent odds) to win between 11 and 13 regular season Big Ten games. The odds of winning 14 or more games are 24 percent, while the odds of winning 10 games or less are 23 percent.
While there are certainly caveats to this analysis, I believe that this result speaks mostly to how very competitive the Big Ten is expected to be this year. If Kenpom’s projections are accurate, they imply that 15 of MSU’s 20 conference games will have a point spread of less than five points.
The Monte Carlo simulation also provides the odds for each team to end the season in at least a tie for first place, which would ensure a share of the regular Big Ten title. Those odds are summarized below in Table 2.
Table 2: Big Ten regular season championship odds based on the results of a 1,000,000 cycle Monte Carlo simulation utilizing preseason Kenpom adjusted efficiency margin data.
As Table 2 shows, Wisconsin is projected to have the best odds to win the regular season title at 38 percent. These odds are over twice as good as the teams with the next best odds: Ohio State (18 percent) and Michigan State (18 percent).
Wisconsin’s huge odds advantage is due in large part to the previously mentioned schedule advantage. If MSU and Wisconsin were to meet on a neutral court, the Badgers would only be projected to be favored by about a point, based on the Kenpom data.
To put this in further perspective, if MSU were to have Wisconsin’s schedule, I calculate that the Spartan’s odds to win the Big Ten regular season title would rise to 26 percent (an eight percentage point improvement). Conversely, if Wisconsin were to play the Spartan’s schedule, their odds to win the Big Ten would fall to 28 percent (a drop of 10 percentage points).
The win distribution data in Table 2 provides some additional hints as to how the Big Ten regular season title race to likely to proceed. The numbers at the bottom of the table give the odds of the final record of the eventual Big Ten champ(s). The most likely outcome is that the Big Ten champ will finish 15-5 (34 percent odds). The odds of the Big Ten champ finishing at 17-3 or better is only nine percent. A 16-4 champ has 20 percent odds, while the odds of the champ(s) finishing at 14-6 are 29 percent.
It should also be noted that the final record of the eventual Big Ten champ is significantly higher than the expected number of wins for any Big Ten team. In other words, the eventual champion(s) will almost certainly need to overachieve relative to the Vegas spread / nominal victory odds.
For example, MSU is only “expected” to win about 12 games. In order to claim the Big Ten title, MSU will likely need “overachieve” by two or three games in order to hang another banner. But, overachieving is exactly what championship teams do. They win more toss-up games than the non-championship teams.
As I mentioned above, there are quite a few caveats to this analysis. Just like any other simulation, the results shown above as only as good as the source data and assumptions. In this case, the biggest source of potential error is in the Kenpom data itself. While I still believe that efficiency data is a gold standard, it is certainly not infallible, especially in the preseason, and especially in 2020. If MSU (or other Big Ten teams) are significantly better (or worse) than Pomeroy’s preseason projections, that team’s odds will obvious either increase or decrease.
In my analysis of college football, I have enough historical data to build in a level of known uncertainty into the calculations. I don’t current have that data for basketball, but I plan to start collecting it. In the future, this should allow for a more accurate prediction of Big Ten basketball odds.
We now know MSU’s odds to win the regular season Big Ten title (just under 20 percent). But, that is not the only Big Ten banner at stake in 2021. The Spartans will also have a shot to hang a banner for winning the Big Ten Tournament at the end of the year.
My Monte Carlo simulation provide more information than just the number of wins for each team and the odds to finish in at least a tie for first place. I also can project the most likely seed for each team in the Big Ten Tournament. Once I have that data, it is also possible to simulate the results of the tournament itself. That will be the subject of the third and final installment in this series. Stay tuned.
While this information is interesting, the real question is what can the data tell us about MSU’s odds to hang a fourth consecutive regular season championship banner in the rafters of Breslin Center? Fortunately, I have just to tools at my disposal to answer that question.
I have described my methodology in the past in some detail, but the basic details are as follows. I start with the data from Ken Pomeroy’s website which tabulates the adjusted offensive and defensive efficiencies of each team. If these numbers are subtracted, it provides an “adjusted efficiency margin”, which correlates very well to the Las Vegas spread.
I have shown previously how the Las Vegas line correlates to the probability that the favored team will win straight up. The odds can be modeled using the the Normal/Gaussian distribution where the mean is the spread itself and the standard deviation is 10 points. I even derived why the standard deviation is 10 points, which is due to the fact that basketball teams usually shoot around 50 percent from the field and college basketball games usually consist of about 70 possessions. For reference, Figure 1 below shows the correlation between the spread and the odds for the favored team to win.
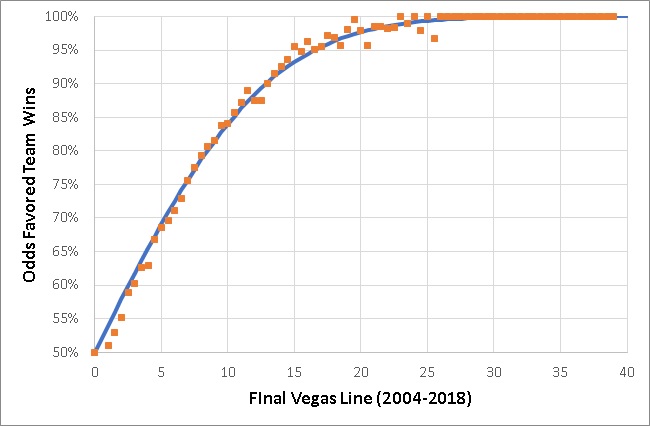
Figure 1: Correlation between the closing Vegas line and the odds that the favored team wins straight up. The best fit line is based on the Normal Distribution where the standard deviation is equal to 10.04 points.
Once there is a method to predict the odds for the outcome of any arbitrary game, it is possible to set up a Monte Carlo simulation to calculate the odds of various full season outcomes. This type of simulation is basically a very large set of virtual coin flips using a random number generator where the “coin” is weighted based on the odds generated from the Kenpom efficiency margin data and the correlation in Figure 1.
In this case, I simulated the entire Big Ten regular season a full 1,000,000 times, because my computer didn’t have anything better to do on a Friday night. The results of this simulation provide a great deal of information, including the expected number of wins for each team, as well as the odds of winning anywhere from zero to 20 conference games. Those data are summarized below in Table 1.
Table 1: Expected win totals and the full win distribution matrix based on 1,000,000 Monte Carlo simulations of the Big Ten season utilizing Kenpom's preseason adjusted efficiency margin data
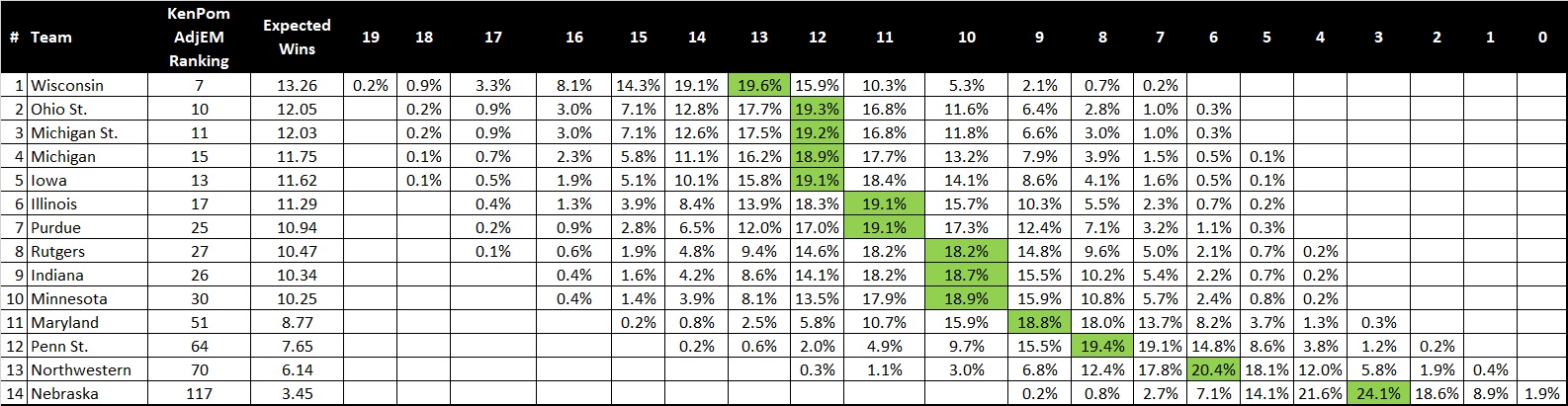
As mentioned in Part One, the preseason Kenpom data suggests a very competitive Big Ten race, with six Big Ten teams in Kenpom’s preseason top 20 and 10 Big Ten teams in the preseason top 30. As such, the top 10 conference teams all have similar expected win totals which range from just over 10 win for Minnesota and Indiana to over 13 wins for Wisconsin.
Not only do the Badgers have the highest preseason Kenpom ranking, but they also have the easiest overall conference schedule. As a result their projected expected win total (13.3) is over a full game higher than the next two teams on the list: Ohio State (12.0) and Michigan State (12.0).
As for MSU, as the win distribution data shows, based on this analysis the Spartans are most likely (53 percent odds) to win between 11 and 13 regular season Big Ten games. The odds of winning 14 or more games are 24 percent, while the odds of winning 10 games or less are 23 percent.
While there are certainly caveats to this analysis, I believe that this result speaks mostly to how very competitive the Big Ten is expected to be this year. If Kenpom’s projections are accurate, they imply that 15 of MSU’s 20 conference games will have a point spread of less than five points.
The Monte Carlo simulation also provides the odds for each team to end the season in at least a tie for first place, which would ensure a share of the regular Big Ten title. Those odds are summarized below in Table 2.
Table 2: Big Ten regular season championship odds based on the results of a 1,000,000 cycle Monte Carlo simulation utilizing preseason Kenpom adjusted efficiency margin data.
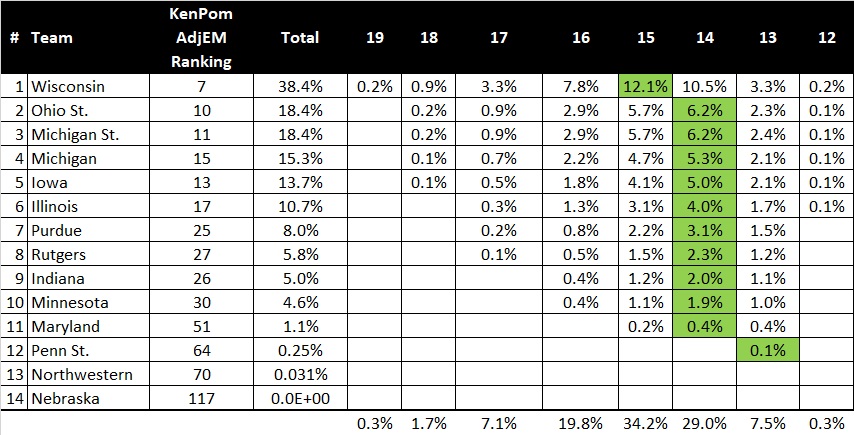
As Table 2 shows, Wisconsin is projected to have the best odds to win the regular season title at 38 percent. These odds are over twice as good as the teams with the next best odds: Ohio State (18 percent) and Michigan State (18 percent).
Wisconsin’s huge odds advantage is due in large part to the previously mentioned schedule advantage. If MSU and Wisconsin were to meet on a neutral court, the Badgers would only be projected to be favored by about a point, based on the Kenpom data.
To put this in further perspective, if MSU were to have Wisconsin’s schedule, I calculate that the Spartan’s odds to win the Big Ten regular season title would rise to 26 percent (an eight percentage point improvement). Conversely, if Wisconsin were to play the Spartan’s schedule, their odds to win the Big Ten would fall to 28 percent (a drop of 10 percentage points).
The win distribution data in Table 2 provides some additional hints as to how the Big Ten regular season title race to likely to proceed. The numbers at the bottom of the table give the odds of the final record of the eventual Big Ten champ(s). The most likely outcome is that the Big Ten champ will finish 15-5 (34 percent odds). The odds of the Big Ten champ finishing at 17-3 or better is only nine percent. A 16-4 champ has 20 percent odds, while the odds of the champ(s) finishing at 14-6 are 29 percent.
It should also be noted that the final record of the eventual Big Ten champ is significantly higher than the expected number of wins for any Big Ten team. In other words, the eventual champion(s) will almost certainly need to overachieve relative to the Vegas spread / nominal victory odds.
For example, MSU is only “expected” to win about 12 games. In order to claim the Big Ten title, MSU will likely need “overachieve” by two or three games in order to hang another banner. But, overachieving is exactly what championship teams do. They win more toss-up games than the non-championship teams.
As I mentioned above, there are quite a few caveats to this analysis. Just like any other simulation, the results shown above as only as good as the source data and assumptions. In this case, the biggest source of potential error is in the Kenpom data itself. While I still believe that efficiency data is a gold standard, it is certainly not infallible, especially in the preseason, and especially in 2020. If MSU (or other Big Ten teams) are significantly better (or worse) than Pomeroy’s preseason projections, that team’s odds will obvious either increase or decrease.
In my analysis of college football, I have enough historical data to build in a level of known uncertainty into the calculations. I don’t current have that data for basketball, but I plan to start collecting it. In the future, this should allow for a more accurate prediction of Big Ten basketball odds.
We now know MSU’s odds to win the regular season Big Ten title (just under 20 percent). But, that is not the only Big Ten banner at stake in 2021. The Spartans will also have a shot to hang a banner for winning the Big Ten Tournament at the end of the year.
My Monte Carlo simulation provide more information than just the number of wins for each team and the odds to finish in at least a tie for first place. I also can project the most likely seed for each team in the Big Ten Tournament. Once I have that data, it is also possible to simulate the results of the tournament itself. That will be the subject of the third and final installment in this series. Stay tuned.